Painstaking Lessons Of Tips About Can Ordinal Data Be Quantitative
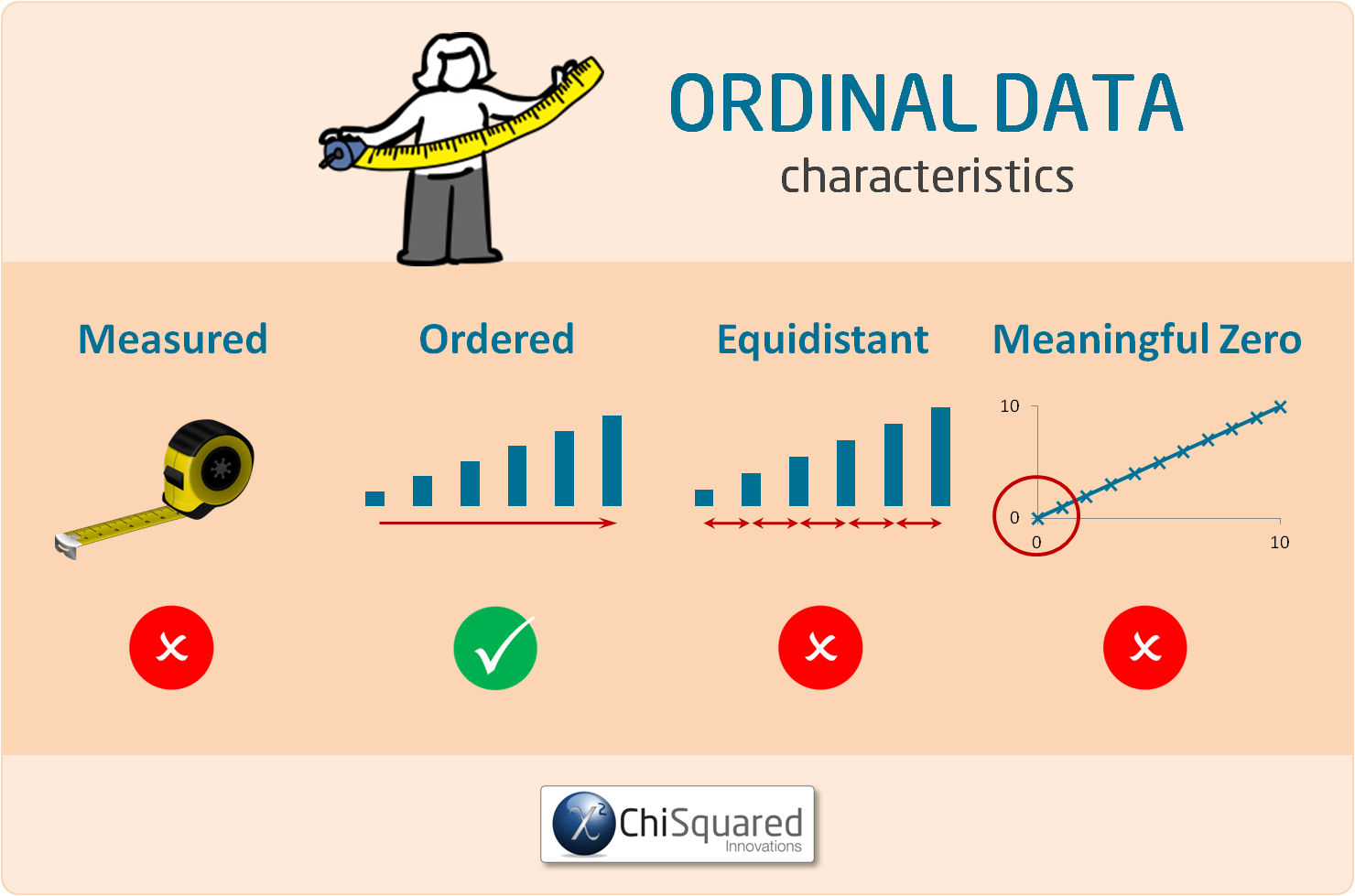
Delving into Data's Depths: When Ordered Categories Meet Numbers
Exploring the Realm Where Qualitative Hints at Quantitative
Have you ever pondered a survey response like "Excellent," "Good," "Fair," "Poor," and wondered if there's a hidden numerical story within those ordered words? It's a common curiosity in the world of research and data analysis: can we truly consider ordinal data, which presents information in a meaningful sequence but lacks consistent intervals, as if it were quantitative data, where the differences between values hold uniform significance?
Consider this: we often assign numerals to these categories (for instance, 1 through 4 for the scale above). The temptation arises to then calculate averages, standard deviations, and even employ statistical tests designed for interval or ratio data. After all, numbers are numbers, aren't they? Well, the journey from ordered categories to quantifiable measurements demands careful thought and often involves some statistical maneuvering. We must remain aware of the inherent limitations of ordinal scales.
The central challenge lies in the unknown and potentially varying distances between the categories. While we understand "Good" is better than "Fair," we don't know the precise degree of that difference. Is the gap between "Excellent" and "Good" the same as the gap between "Poor" and "Fair"? Not necessarily! This absence of consistent intervals is the fundamental distinction between ordinal data and truly quantitative data.
Despite this complexity, analysts and researchers frequently use techniques to analyze ordinal data in a quantitative manner. This might involve assuming equal intervals (though this assumption should always be stated clearly and ideally examined), or employing statistical methods that are more resilient to violations of interval scale assumptions. The underlying reason often stems from a desire to conduct more powerful statistical analyses and to summarize the data in a more easily understandable numerical format. But it's wise to tread carefully here!
The Lure and the Labyrinth of Quantification
Examining When Numbers Might Oversimplify the Narrative
The attraction of treating ordinal data as quantitative is quite understandable. It unlocks a broader spectrum of statistical tools, potentially leading to more sophisticated analyses and richer insights. Imagine being able to state, "On average, satisfaction levels increased by 0.6 points following the service improvement." This sounds more tangible than simply saying more people reported higher satisfaction.
However, this convenience carries potential risks. If the actual differences between the ordinal categories are not uniform, then treating the data as quantitative can produce misleading results and flawed conclusions. Calculating an average of "Excellent" (4) and "Poor" (1) to get 2.5 might lack real-world meaning if the psychological distance between those categories isn't consistent.
Furthermore, applying statistical tests intended for interval or ratio data to ordinal data can sometimes violate the fundamental requirements of those tests, potentially leading to inaccurate probability values and incorrect interpretations. It's akin to using a screwdriver as a hammer — it might work in a pinch, but it's not the right tool for the job and could cause damage (in this case, to the validity of your analysis).
Therefore, while the urge to quantify ordinal data is strong, it's essential to balance the potential advantages against the dangers of misinterpretation. Always consider the fundamental nature of your data and the assumptions underlying the statistical methods you choose. A healthy sense of skepticism and a clear grasp of the limitations are your most valuable assets in this endeavor.
Approaches to Bridging the Divide
Techniques for Extracting Numerical Meaning Responsibly
Despite the inherent distinctions, there are ways to glean meaningful quantitative insights from ordinal data without completely disregarding its categorical essence. One common approach involves using non-parametric statistical methods, which make fewer assumptions about the underlying distribution of the data and are more appropriate for ordinal scales. Examples include the Mann-Whitney U test, the Kruskal-Wallis test, and Spearman's rank correlation coefficient.
Another useful strategy is to visualize the data in ways that emphasize the ordered categories, such as stacked bar charts or frequency distributions. This allows for a more nuanced understanding of the data than simply examining averages and standard deviations. You can observe trends and shifts in the distribution across different groups or time periods without assuming equal intervals between the categories.
Moreover, researchers sometimes employ techniques like assigning scores based on theoretical or empirical justifications. For instance, in certain psychological assessments, there might be established reasons for assigning specific numerical values to ordinal categories based on underlying psychological constructs. However, this process should be transparent and supported by a clear rationale for the assigned values.
Ultimately, the crucial element is to be thoughtful and transparent in your analytical process. Acknowledge the limitations of treating ordinal data as quantitative, justify your methodological choices, and interpret your findings with caution, always remembering the original categorical nature of the data. Think of it as translating a subtle language — you can convey the general meaning, but some of the finer points might be lost in the numerical translation.
The Google Lens: Enhancing Visibility and Connection
Crafting Content That Resonates with Search Systems and People
From the perspective of Google Discover and Search, creating content that thoughtfully explores the intricacies of data analysis, such as the question of ordinal versus quantitative data, can be exceptionally valuable. Search engines prioritize informative, well-organized content that directly addresses specific user inquiries and provides comprehensive explanations. By clearly explaining the concepts, potential pitfalls, and recommended practices, you are producing content that is likely to be recognized as authoritative and helpful.
Employing clear headings (like our `
` and `` tags), concise paragraphs (`
`), and incorporating relevant keywords (such as "ordinal data," "quantitative data," "statistical analysis," "Likert scale," "levels of measurement") assists search engines in understanding the topic and connecting it with relevant user searches. Furthermore, engaging with the topic in a nuanced and considerate manner, as we've aimed to do here, can maintain reader interest and encourage longer engagement times, which is another positive indicator for search algorithms.
Consider the FAQs we will include — these directly address common questions users might have, offering quick and accessible answers. This not only improves the user experience but also increases the likelihood of your content appearing in featured snippets or answer boxes on Google Search. By anticipating user needs and providing thorough and easy-to-understand information, you are essentially optimizing your content for both algorithmic understanding and human comprehension.
Therefore, when developing your content, whether it concerns data analysis or any other subject, strive for clarity, depth, and a user-focused approach. By addressing complex topics in an accessible way and structuring your content logically, you are not merely improving your chances of higher rankings; you are also providing genuine value to your audience. And that, in the end, is what both Google and your readers truly appreciate.
Frequently Asked Questions
Addressing Your Inquiries (Hopefully with a Touch of Lightness)
Alright, let's tackle some of those persistent questions that might be occupying your insightful minds!
Q: So, is treating ordinal data as quantitative always a misstep? Are there *any* exceptions?
It's generally a path to tread with caution, but there are situations where it's practiced, particularly with large datasets where the central limit theorem might offer some statistical reassurance. However, transparency about your assumptions is paramount, and you should always consider the potential for misinterpretation. Think of it like bending the rules slightly — sometimes it might seem okay, but it's important to understand the potential consequences and have a good reason for doing so.
Q: What are some important terms to remember when writing about this to improve search visibility?
That's a smart question! Consider the phrases someone searching for this information might use. Terms like "ordinal data vs quantitative data," "treating ordinal data as interval data," "analyzing Likert scale data," "non-parametric statistical tests," "levels of data measurement," and "statistical methods for ordinal data" are all valuable to include naturally within your content. Think of them as helpful signposts that guide both search engines and readers to your insightful explanations.
Q: If directly treating ordinal data as quantitative isn't always ideal, what are some better approaches? Any helpful techniques?
Indeed! Focusing on non-parametric methods is a solid starting point, as these techniques don't rely on strict assumptions about your data's distribution. Visualizations are also incredibly helpful — bar charts, histograms, and box plots can reveal important patterns without forcing the data into a quantitative framework. And don't underestimate the power of simply examining the frequencies and percentages within each category. It might not seem as statistically advanced as some tests, but it can provide very meaningful insights. It's about appreciating the unique characteristics of your data rather than trying to force it into a one-size-fits-all analytical box.